A4Snow
A4Snow is a Decision Support Tool able to translate flood and snow forecasts into road network performance impact specifically targeted at enabling self-response of the logistic platforms of the food distribution companies during severe weather events. It has been tested during the 2019 snow season.
A4Snow shows a real-time estimation of the extent of the affected area and the impact on the circulation conditions, which are then translated into the impact on the network capacity, by cross-correlating the weather forecasts (such as level of snow, precipitation, etc.). Afterwards it simulates and evaluates different transport network scenarios. This tool is conceived as support to companies dealing with distribution tasks.
Pilot Site: Catalonia
Case study: A4Snow (ANYWHERE for Snow)
Status: Under operational demonstration since November 2018
Developer and implementor: RINA-C and CRAHI-UPC (Centre de Recerca Aplicada en Hidrometeorologia-Universitat Politècnica de Catalunya )
End-users: Food distribution companies and CECAT (Centre de Coordinació Operativa de Catalunya) belonging to INTC (Departament d’Interior-Generalitat de Catalunya)
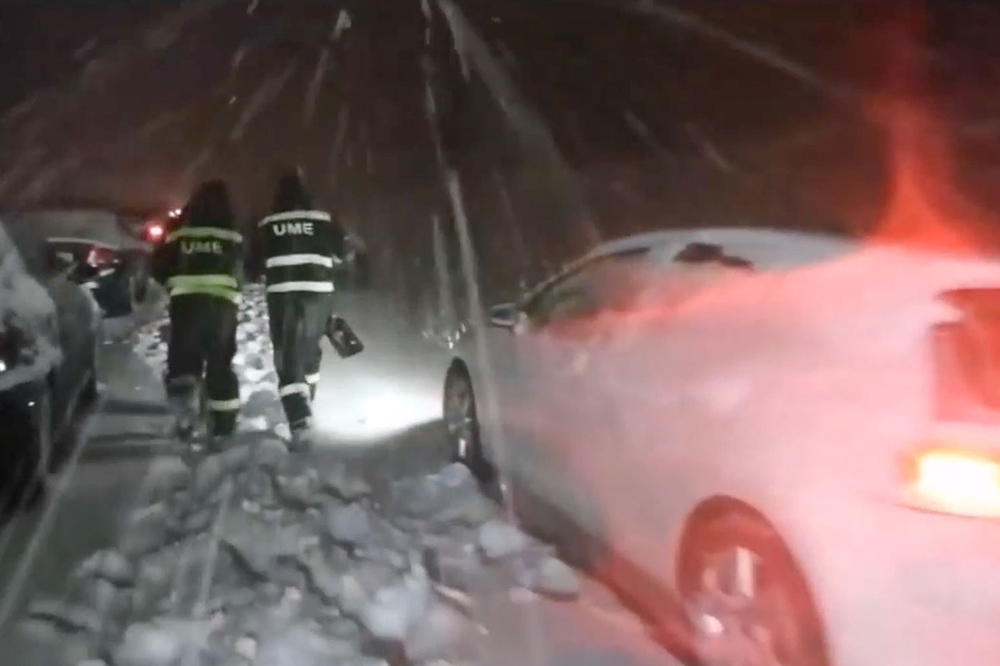
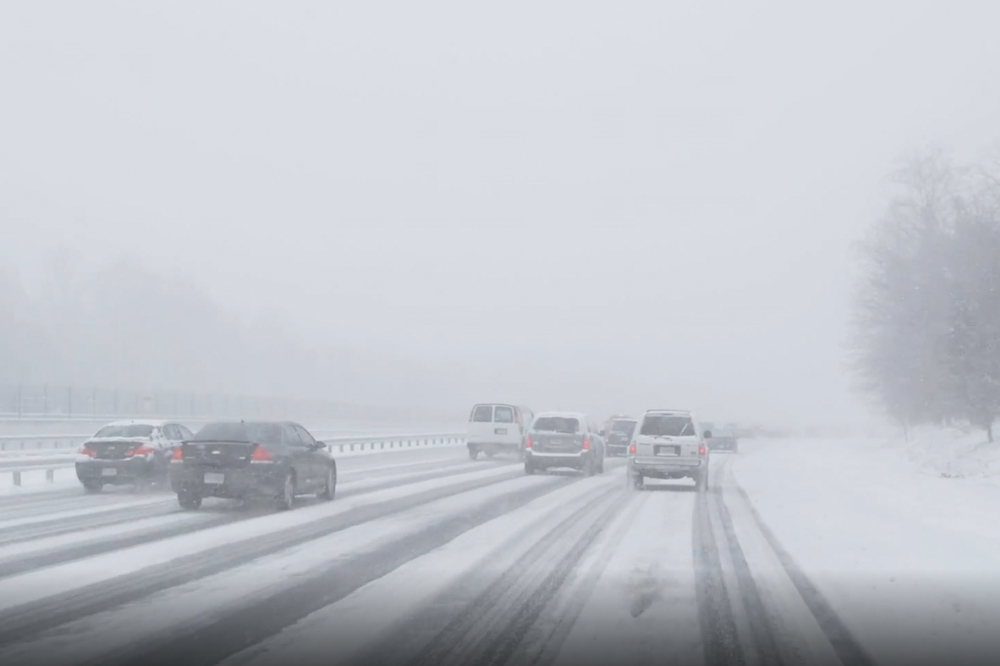
A4Snow
A4Snow is a Decision Support Tool able to translate flood and snow forecasts into road network performance impact specifically targeted at enabling self-response of the logistic platforms of the food distribution companies during severe weather events. It has been tested during the 2019 snow season.
A4Snow shows a real-time estimation of the extent of the affected area and the impact on the circulation conditions, which are then translated into the impact on the network capacity, by cross-correlating the weather forecasts (such as level of snow, precipitation, etc.). Afterwards it simulates and evaluates different transport network scenarios. This tool is conceived as support to companies dealing with distribution tasks.
Pilot Site: Catalonia
Case study: A4Snow (ANYWHERE for Snow)
Status: Under operational demonstration since November 2018
Developer and implementor: RINA-C and CRAHI-UPC (Centre de Recerca Aplicada en Hidrometeorologia-Universitat Politècnica de Catalunya )
End-users: Food distribution companies and CECAT (Centre de Coordinació Operativa de Catalunya) belonging to INTC (Departament d’Interior-Generalitat de Catalunya)
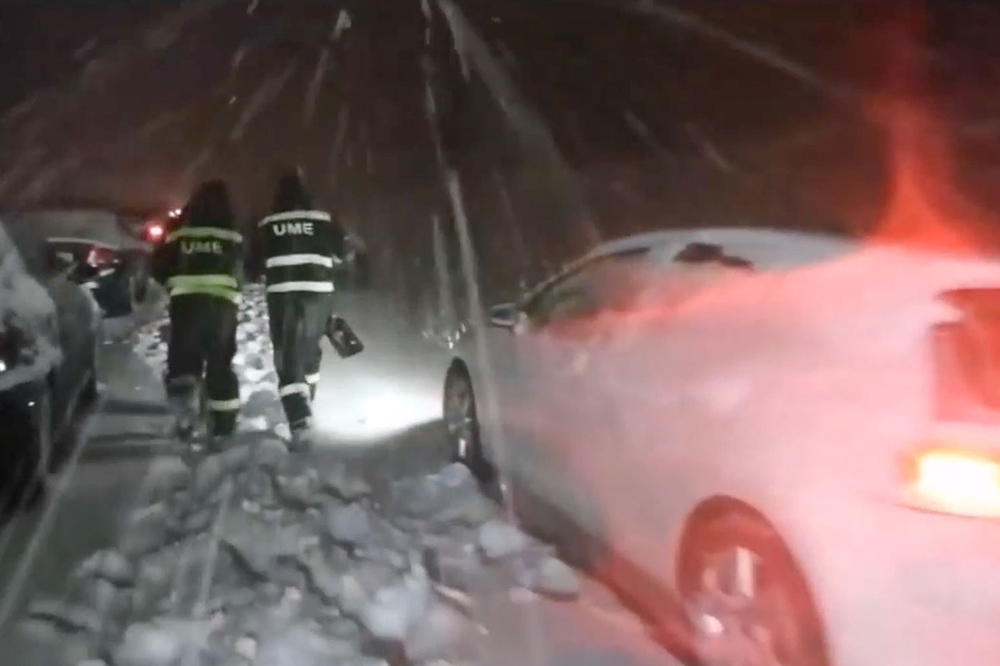
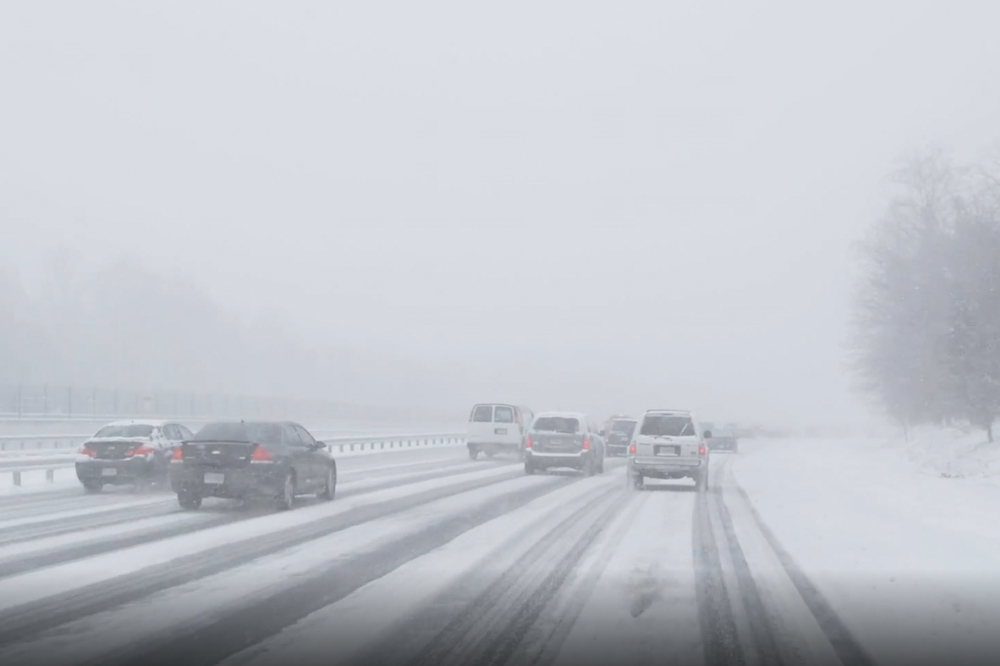
It is customized to the needs of food distribution logistics platforms, it is integrated with the ANYWHERE Multi-Hazard Early Warning System (MH-EWS) and, in addition, on the Catalonia Pilot Site, demonstrating its potential transferability to other applications.
It is customized to the needs of food distribution logistics platforms, it is integrated with the ANYWHERE Multi-Hazard Early Warning System (MH-EWS) and, in addition, on the Catalonia Pilot Site, demonstrating its potential transferability to other applications.
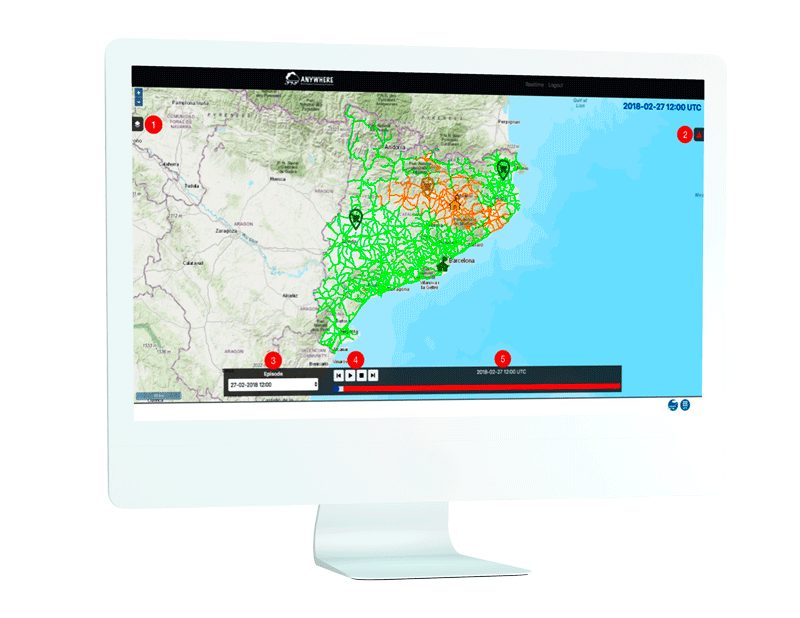
Main functionalities:
Collects the forecasts available in the ANYWHERE Multi-Hazard Early Warning System (MH-EWS) to translate weather observations and forecasts to the impact on roads and circulation conditions.
Provides high-resolution forecasts of the road and circulation conditions and indications for logistics-related companies useful to find or choose the best route.
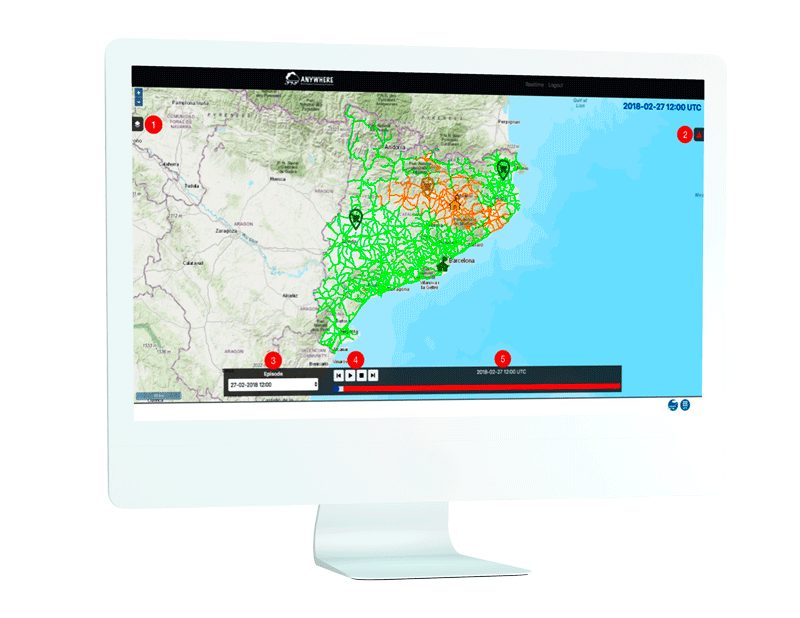
Main functionalities:
Collects the forecasts available in the ANYWHERE Multi-Hazard Early Warning System (MH-EWS) to translate weather observations and forecasts to the impact on roads and circulation conditions.
Provides high-resolution forecasts of the road and circulation conditions and indications for logistics-related companies useful to find or choose the best route.
Citizen participation:
The initiative @carreteranevada consists of monitoring the state of roads affected by snowfalls with the help of citizen participation.
People who find snowy roads can send geolocalized photographs of the affected roads through Twitter.
The photographs received from the different snow episodes that occurred during the winter will allow to validate and improve the accuracy of the current forecasting model.
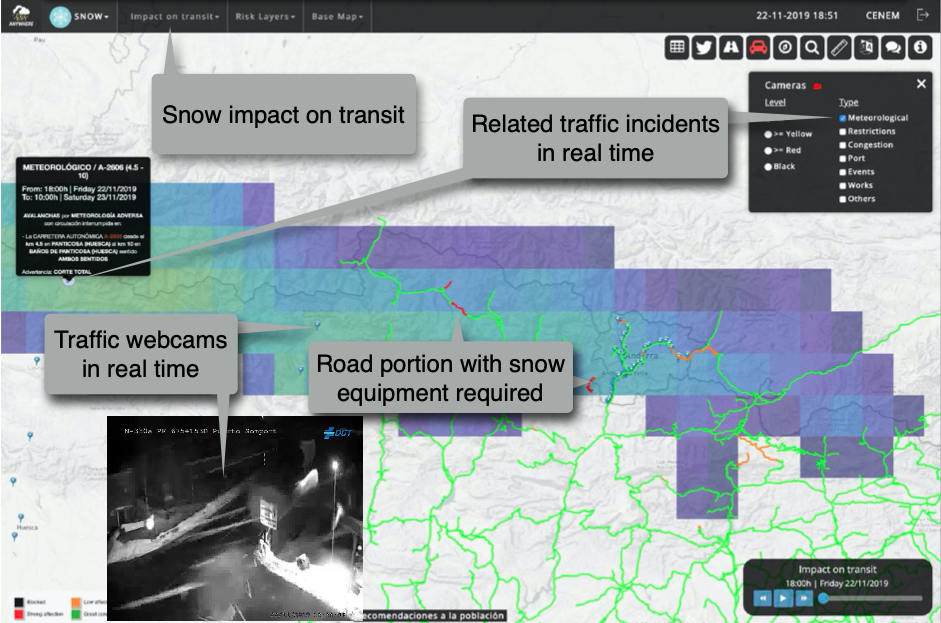